Revolutionising ETL: Unlocking Business Intelligence with Next Generation Data Transformation
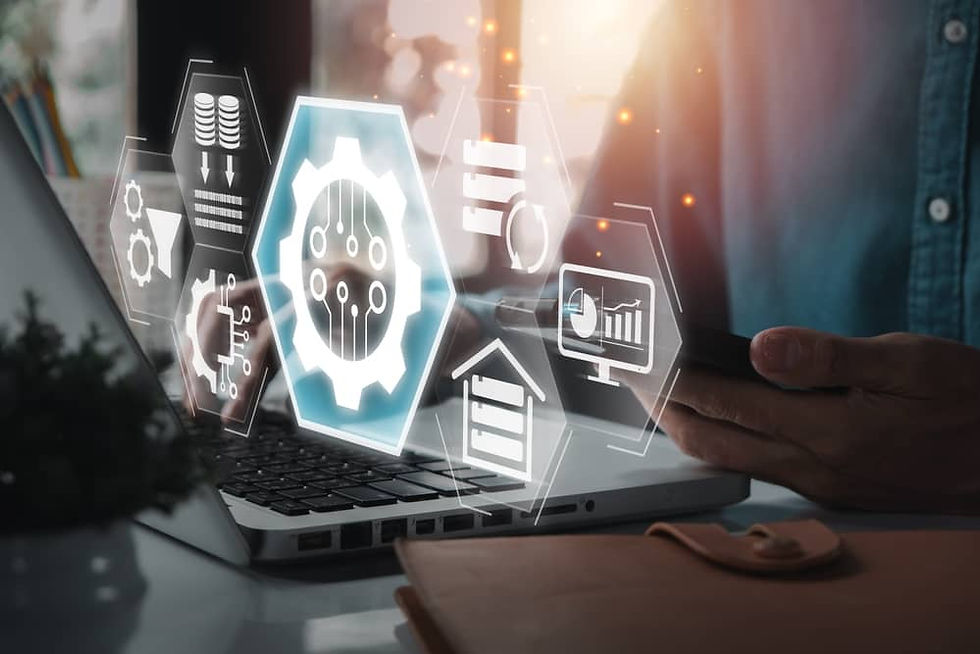
Executive Summary
In a landscape defined by rapid technological advancement, the ability to manage, process and derive value from data has become a strategic imperative. Organisations that fail to optimise their ETL (Extract, Transform, Load) processes risk falling behind in an increasingly data driven world.
This white paper explores the essential role of ETL in transforming raw, disparate data into refined, actionable insights. With businesses leveraging data to enhance intelligence, improve decision making and maximise ROI, modernising ETL frameworks is no longer optional—it is critical. Efficient ETL processes not only enhance business intelligence but also fortify data governance and streamline operations.
We delve into the full ETL lifecycle, addressing the complexities of managing vast and diverse data sources. This includes examining the common challenges businesses face, from scalability limitations to governance and integration hurdles. More importantly, we highlight cutting edge technologies such as Databricks and Apache Spark that are revolutionising ETL, enabling organisations to accelerate data processing, reduce costs and unlock new opportunities.
By adopting best practices and leveraging next generation ETL strategies, enterprises can future proof their data infrastructure, ensuring they remain competitive and ready to harness the full potential of their data assets.
Get a free recap to share with colleagues
What is Lorem Ipsum?
Lorem Ipsum is simply dummy text of the printing and typesetting industry. Lorem Ipsum has been the industry's standard dummy text ever since the 1500s, when an unknown printer took a galley of type and scrambled it to make a type specimen book. It has survived not only five centuries, but also the leap into electronic typesetting, remaining essentially unchanged.

Lorem Ipsum is simply dummy text of the printing and typesetting industry. Lorem Ipsum has been the industry's standard dummy text ever since the 1500s, when an unknown printer took a galley of type and scrambled it to make a type specimen book. It has survived not only five centuries, but also the leap into electronic typesetting, remaining essentially unchanged.
What is ETL and Why is it Important?
ETL is a foundational data integration process that enables organisations to consolidate, refine and structure vast amounts of raw information into meaningful insights. It serves as the backbone of enterprise data management, ensuring that data is accurate, accessible and optimised for business intelligence, analytics and operational decision making.
A well architected ETL pipeline enables efficient data migration, cleansing and enrichment, transforming fragmented datasets into a unified source of truth. This not only enhances reporting accuracy but also fuels AI driven decision making and predictive analytics, making ETL optimisation a key enabler of business success.

Extract, Transform, Load: The Three Pillars of ETL
ETL is a structured, step by step process where data moves through three critical stages:
Step 1: Extraction
The extraction phase involves retrieving data from multiple sources, consolidating disparate datasets and preparing them for further processing. These sources may include on premise databases, CRM systems, ERP platforms, structured and unstructured files, cloud applications and IoT data streams.
Since raw data can exist in various formats and structures, it must be standardised and organised based on key attributes such as timestamps, categories and source systems. The complexity of extraction varies depending on data types, volume and source diversity, requiring careful planning to ensure a seamless transition into the transformation phase.
Key Extraction Steps:
Retrieve and consolidate data from relevant sources
Standardise data formats for consistency
Step 2: Transformation
The transformation phase is where raw data undergoes refinement to ensure it aligns with business requirements. This process involves data cleansing, reformatting, enrichment and validation before loading it into the target system.
Transformation can range from applying simple data mapping rules to executing complex computations, standardisation techniques and AI driven anomaly detection. A robust transformation process ensures data integrity, enhances compatibility across systems and facilitates meaningful analysis.
Key Transformation Steps:
Convert data to align with business logic and compliance standards
Reformat datasets for seamless compatibility
Cleanse data by sorting, filtering, deduplicating and resolving inconsistencies
Step 3: Loading
The final step involves loading the transformed data into a destination system, such as a data warehouse, data lake, or real time analytics platform. This can be achieved through either bulk loading or incremental SQL inserts, depending on business needs.
Bulk loading is optimal for handling large datasets efficiently, whereas row by row SQL inserts are ideal for ensuring data integrity and validation checks. A well structured loading process ensures that data remains accessible, queryable and optimised for real time analytics and decision making.
Key Loading Steps:
Load well structured, cleansed data into the target system
Optimise for performance and query efficiency
Challenges in ETL Optimisation
Implementing reliable ETL processes in today’s world of vast and complex data is a demanding task. Organisations must navigate multiple obstacles to ensure their ETL frameworks operate efficiently, scale effectively and deliver accurate insights. Below are some of the most common challenges businesses face when optimising ETL workflows.
Managing Data Volume
With data growing at an unprecedented rate, ETL processes must handle increasing volumes efficiently. Some business systems only require incremental updates, while others need full data reloads for each cycle. The ability to scale processing power to accommodate both structured and unstructured data is essential for maintaining performance and reliability.
Ensuring Data Speed and Real Time Processing
In today’s fast paced business environment, real time data connectivity is crucial for timely insights and decision making. As business intelligence shifts toward real time analytics, organisations must update data warehouses and marts frequently. This requires a hybrid approach that integrates batch processing with real time streaming technologies such as Apache Kafka or Spark Streaming, ensuring that insights remain accurate and actionable.
Integrating Data from Disparate Sources
As organisations adopt more complex digital ecosystems, they must extract data from an ever growing number of sources, including databases, APIs, cloud applications, IoT devices and unstructured repositories. ETL tools must be highly adaptable, capable of integrating with various systems seamlessly and handling different data formats to enable smooth data consolidation.
Transforming Data for Distinct Targets
Different business intelligence systems, data warehouses and data marts have unique data structures, requiring extensive transformations. ETL processes must support complex transformations, including aggregations, computations and statistical processing, while also accommodating specific business intelligence functions like slowly changing dimensions (SCDs). Additionally, resolving multiple data sources and keys is critical to maintaining data integrity and consistency across integrated systems.
Overcoming Skill Shortages in ETL Implementation
A lack of specialised technical skills is a significant barrier to ETL optimisation. Many enterprises struggle to find data engineers proficient in modern ETL frameworks, leading to underutilisation of available tools. Poorly managed ETL processes result in data inconsistencies, unreliable analytics and increased security risks. Investing in workforce training, automation and AI powered ETL solutions can mitigate these challenges, ensuring organisations maximise the potential of their data assets.
Practical Steps for ETL Optimisation
Effective ETL optimisation is the key to unlocking the full potential of enterprise data. A well structured ETL framework ensures accurate, timely and actionable insights while reducing operational inefficiencies. However, achieving optimal ETL performance requires a strategic approach that incorporates skilled personnel, well defined processes, cutting edge technologies and robust governance structures.
This chapter explores the practical steps organisations can take to streamline their ETL workflows, enhance data management and maximise the value of their data assets. By focusing on four core pillars: People, Process, Technology and Governance, businesses can ensure that their ETL pipelines are resilient, scalable and aligned with their strategic goals.

People
A successful ETL strategy depends on having the right team with well defined roles, responsibilities and skill sets. Organisations should invest in hiring and training data engineers proficient in modern ETL technologies such as Databricks and Apache Spark. Regular up-skilling ensures that employees can leverage these tools effectively and stay ahead of industry trends.
Process
Optimising ETL processes requires a well structured workflow that includes automation, validation and continuous performance tuning. Organisations should implement automated validation checks at each stage of the ETL pipeline to reduce errors and improve data quality.

Technology
Leveraging advanced ETL platforms is crucial for streamlining data processing and improving efficiency. Platforms such as Databricks provide scalable, distributed computing capabilities that allow organisations to process large datasets in real time.
Governance
A strong data governance framework ensures data integrity, security and compliance with industry regulations. Organisations should establish clear policies for data access, security and audit control, ensuring that sensitive information is protected.
Case Study: Optimising ETL for Marks & Spencer
Background
Marks & Spencer, one of the UK's most recognised retail brands, embarked on a data transformation initiative to enhance its analytics capabilities. The company needed to integrate a newly onboarded data quality tool into its Synapse Analytics environment. To achieve this, an efficient ETL pipeline was required to ensure seamless data migration while maintaining data integrity and governance.
Cloudaeon was engaged to implement and support the end to end ETL process using Prophecy, a low code data engineering platform, alongside Airflow and Databricks.
Challenges
The project presented a critical deadline: migrating data from the new data quality tool into Synapse Analytics had to be completed within two weeks before the existing data quality tool was decommissioned. The team needed a streamlined approach to implementing Prophecy while ensuring minimal disruption to existing analytics workflows.
Technologies Used
Prophecy: A low code data engineering platform for visual ETL pipeline development.
Apache Airflow: Workflow orchestration for managing the end to end data pipeline.
GitHub Actions: Automation for version control and deployment.
Databricks: Compute infrastructure for executing transformations and data processing.
Solution
Cloudaeon designed and deployed an efficient ETL pipeline leveraging Prophecy’s low code interface to transform and migrate data. Airflow orchestrated Prophecy generated pipelines to ensure structured, dependent execution. Databricks was used for executing Prophecy generated wheel (.wbl) files, ensuring scalability and performance.
This approach not only accelerated development but also eliminated the need for complex custom ingestion frameworks, reducing development time and operational overhead.
Outcome
Marks & Spencer successfully migrated and validated data between the old and new data quality tools within the set deadline. The integration of Prophecy and Airflow enabled a fast, reliable ETL build, reducing deployment time to just two weeks. By bypassing legacy ingestion frameworks, the project delivered a scalable, future proof data pipeline that streamlined analytics operations.
This case study highlights how Cloudaeon’s expertise in ETL modernisation helped Marks & Spencer achieve a seamless data transition, reinforcing the importance of innovative, agile ETL solutions in the retail sector.
Common Pitfalls in Legacy ETL Pipelines
You’re Not Alone: The Challenges of Legacy ETL Systems
Many organisations transitioning from legacy ETL systems face similar obstacles. Even after adopting advanced solutions like Databricks and Apache Spark, enterprises often struggle to realise their full benefits. These challenges can stem from inefficiencies in processes, gaps in expertise and underutilisation of available technology. If your business encounters roadblocks in its ETL transformation journey, know that you are not alone; these issues are common, but they can be resolved with the right strategy and expertise.
Underutilised Features
Many enterprises invest in powerful ETL platforms like Databricks and Apache Spark, yet fail to fully harness their capabilities. Instead of leveraging features such as in memory processing, auto scaling clusters and built in machine learning libraries, organisations often use them as mere replacements for traditional SQL based data processing. This underutilisation results in increased costs and missed opportunities for performance optimisation.
Skill Gaps and Training Deficiencies
While SQL remains a staple for data transformation, it does not directly translate to Spark’s distributed computing model. Engineers without Spark specific training struggle with optimising queries, leading to inefficient data processing and slow performance. Additionally, PySpark (though powerful) is still evolving, requiring teams to continually update their skill sets to make full use of its latest enhancements.
Process Inefficiencies and Lack of Standardisation
Without standardised ETL processes, data engineers often find themselves “reinventing the wheel.” Ad hoc solutions, inconsistent pipeline structures and a lack of documentation create operational bottlenecks. This lack of standardisation not only slows development but also complicates governance, making compliance and auditing more challenging.
AI Implementation Barriers
Many organisations want to incorporate AI into their analytics strategy but find themselves held back by ETL limitations. Data transformation inefficiencies, lack of real time streaming capabilities and poor pipeline orchestration hinder AI model training and deployment. Without well optimised ETL, AI driven insights remain an aspiration rather than a reality.
Shortage of Spark Engineers
Apache Spark expertise is in high demand and finding skilled engineers is a challenge. This shortage places excessive pressure on existing teams, leaving them overburdened and unable to focus on strategic initiatives. Meanwhile, data analysts, who often depend on clean and timely data, remain underutilised due to delayed or inconsistent ETL processes.
The Cloudaeon Approach to ETL Modernisation
At Cloudaeon, we help organisations break free from these common pitfalls. By providing structured ETL optimisation strategies, comprehensive training programs and scalable solutions leveraging Databricks and Apache Spark, we ensure businesses can fully capitalise on their data assets. Our expertise in automation, AI driven transformation and governance ensures that enterprises move beyond legacy ETL limitations and into a future of seamless, intelligent data management.

Conclusion & Recommendations
The Future of ETL is Automated, Collaborative and AI Powered
The evolution of ETL has reached a turning point. Legacy methods can no longer keep up with the demands of real time analytics and AI driven decision making.
A low code, AI powered ETL solution built for Apache Spark is the key to unlocking efficiency, automation and speed.
Key Recommendations for Data Driven Enterprises
Adopt an AI Copilot for ETL: Automate mundane, repetitive tasks, allowing data engineers to focus on innovation.
Leverage Low Code for Faster Deployment: Reduce development time with visual workflows that integrate seamlessly with Apache Spark.
Maximise Automation: Use AI to detect anomalies, optimise workflows and streamline data transformations.
Enable Collaboration: Democratise ETL development by making it accessible to data analysts and business users.
Scale with Cloud and Apache Spark: Leverage server-less execution and auto scaling to handle enterprise level data workloads efficiently.
Final Thoughts
Enterprises that fail to modernise their ETL strategies risk falling behind. By embracing a low code, AI powered ETL platform, businesses can automate workflows, optimise data pipelines and accelerate time to value.
Cloudaeon empower organisations to transition from legacy ETL systems to next generation, automated and AI driven data transformation solutions.
To help businesses take the next step, we offer a 72 Hour Lightning Consultation. A rapid, focused assessment designed to identify inefficiencies, streamline processes and build a strategic roadmap for ETL optimisation.

In only three days, our ETL optimisation experts will evaluate your data pipeline development, technology landscape, workforce integration and automation potential.